How AI-Driven Optimization is Different
Experimentation revolves around validating hypotheses, enhancing user experiences, and unearthing insights that drive business outcomes.
A/B testing stands as a prevalent strategy for experimentation and optimizing conversion rates. However, given its intricacies and narrow focus, organizations require assistance to fully leverage its potential.
Artificial Intelligence simplifies the process of creating, deploying, refining, and evaluating A/B tests, empowering organizations to achieve their objectives with greater speed and efficiency.
What is A/B Testing?
In the realm of customer experience, A/B testing offers a method to compare two different versions of a digital interaction point to determine which yields superior results.
Consider the scenario where you aim to enhance conversions on your homepage.
An elementary illustration of A/B testing involves dividing website traffic evenly into two groups and presenting each group with a slightly varied homepage experience to gauge which generates more conversions. For instance, this could involve altering the color of a call-to-action (CTA) button in the hero section of your website from white to red.
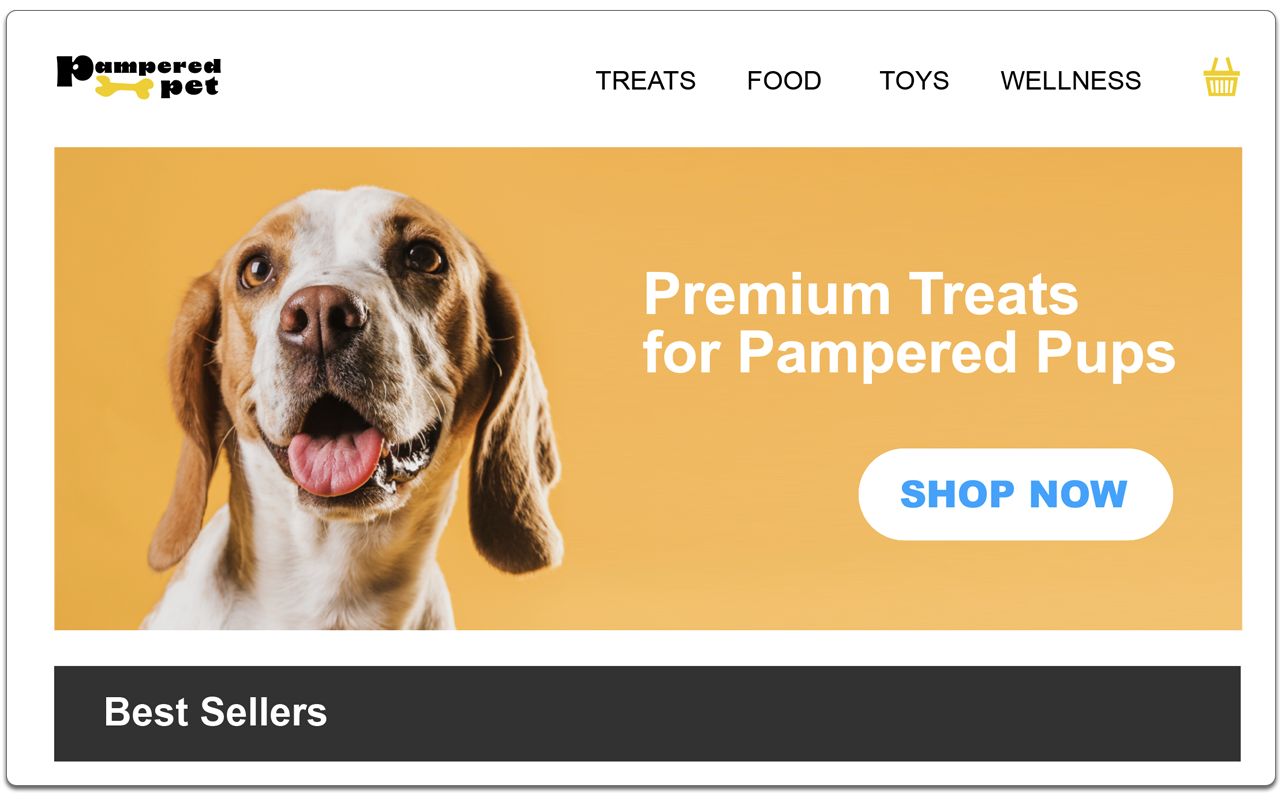
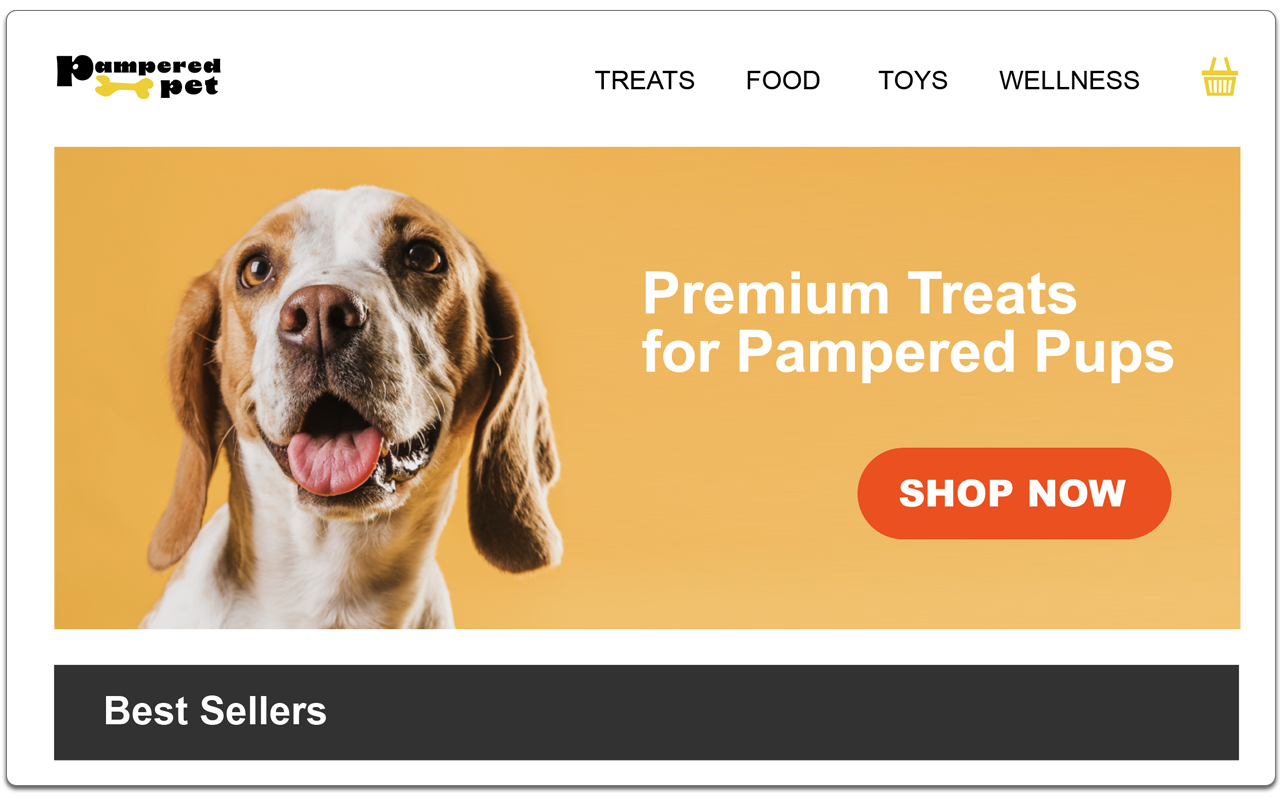
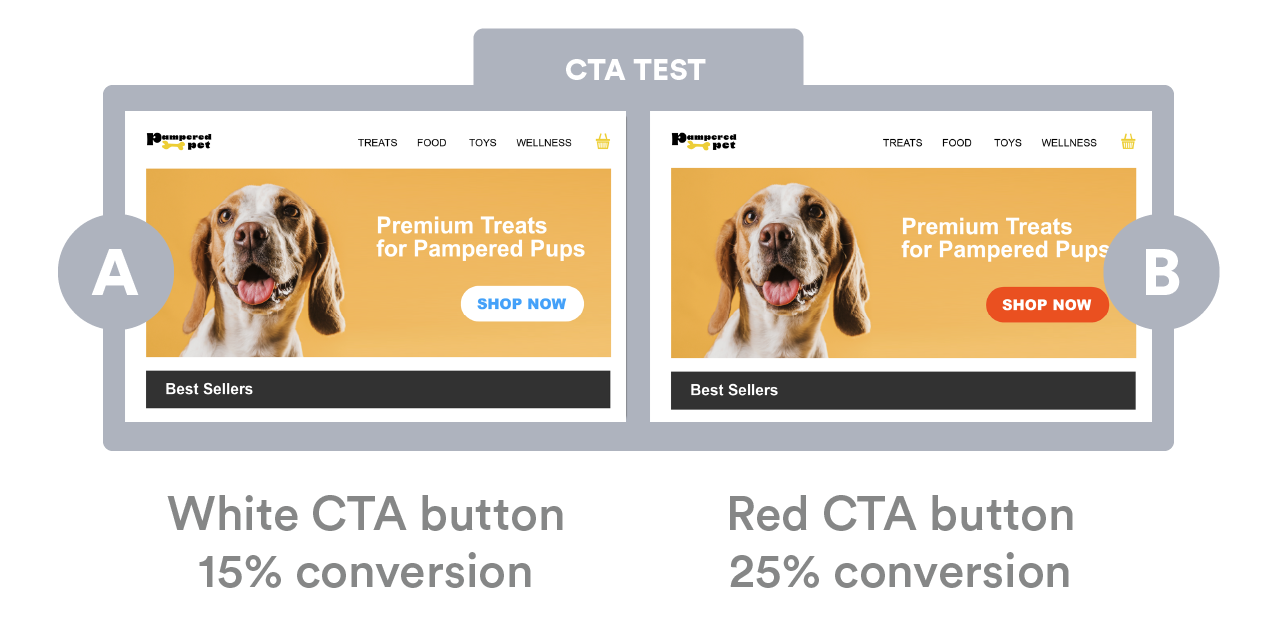
Limitations of A/B Testing
A/B tests are simple to execute, which is why numerous marketing and product teams are drawn to them. However, they lack efficiency:
- Depth: While testing A versus B is beneficial for isolated alterations, such as the color of a CTA button, it often fails to capture the full complexity of user behavior. The impact on conversions is frequently influenced by numerous smaller adjustments across various pages and touchpoints. In such cases, a more comprehensive approach is necessary. Rather than merely comparing two variations, a more insightful experiment would involve testing A versus B versus C versus D versus E versus F, and possibly beyond. Alternatively, exploring combinations of changes, such as A with B and C versus A with D and F, can provide a richer understanding of user preferences and behaviors.
- Velocity: For websites experiencing substantial traffic, abbreviated testing durations may be feasible. However, the reality is that many organizations lack this advantage. In such cases, A/B tests might need to be conducted over extended periods, potentially spanning months, before yielding statistically significant results. Additionally, the rigidity of A/B testing poses challenges when it comes to making mid-course adjustments based on insights or identified flaws in the user experience. Unlike more flexible approaches, A/B testing typically requires starting anew with each iteration, making it cumbersome to implement immediate course corrections.
- Lack of personalization: A/B tests are commonly referred to as split tests because they divide traffic evenly to gain insights. However, the challenge with 50/50 testing lies in its inability to account for the diverse preferences within your audience. Once an experience is deployed, all users receive the same version, making it challenging to gauge how the 50/50 split will perform across your entire audience, particularly within specific segments. For instance, did your split test differentiate between frequent shoppers and first-time visitors? Achieving genuine personalization demands more extensive testing and thorough analysis before implementing targeted rules for tailored experiences.
- Rigid findings: Although A/B testing appears simple, its underlying statistical principles are inflexible. A/B testing relies on Frequentist statistics, which derive conclusions from sample data by focusing on the frequency or proportion of the data. In simpler terms, it’s akin to declaring, “We’ve hit the target. Henceforth, this is the solution.” However, user behaviors can swiftly evolve, and the solution to your challenges may change just as rapidly. Without a more adaptable experimental approach, once the findings from your A/B test lose relevance, you’re essentially starting over from scratch.
Running multiple tests within the same funnel or on the same page is generally discouraged because they can significantly influence each other’s outcomes. Consequently, due to the plethora of constraints mentioned earlier, most brands can only execute a limited number of tests annually. Moreover, when there’s a need to optimize numerous touchpoints across various platforms such as checkout pages, display ads, landing pages, and email marketing — essentially anywhere involving copy, images, or placement — deriving meaningful business impact from A/B testing can seem like an insurmountable challenge.
However, despite the challenges, experimentation remains crucial for mitigating risks and making data-driven decisions. Fortunately, the disruption caused by AI has expanded the possibilities within A/B testing.
AI-led Optimization
In essence, leveraging AI for experimentation empowers brands to achieve greater results more efficiently.
With AI, marketers can explore a significantly larger array of ideas within the same timeframe as traditional A/B testing. Moreover, AI facilitates testing across entire funnels rather than focusing solely on individual pages. Additionally, AI simplifies the process of creating dynamically updating experiences with an unlimited number of versions, while simultaneously enabling personalized experiences tailored to specific audience segments.
- Continuous optimization: Your experiences can achieve "self-optimization" by simultaneously targeting audiences, segments, or general audiences across multiple touchpoints. Unlike A/B testing, AI continuously iterates on running experiments in real-time: eliminating underperforming ideas and seamlessly adding or removing new variants without the need to start and stop an experiment. For instance, if your top-performing combination boosts orders but lowers the average units or items per order, AI can automatically introduce a variant aimed at encouraging higher cart items without requiring a separate test.
- Scale: Traditional A/B testing purists frequently lament the inability to conduct a sufficient number of quality experiments. From brainstorming ideas to deployment, analysis, and optimization, the A/B testing process entails significant time investment. However, integrating AI into this process can alleviate these challenges, saving valuable organizational time. AI has the capability to learn from experiment outcomes, automatically discerning what works and what doesn't, and subsequently recommending the most effective UX actions. Additionally, AI aids in project development, assisting with tasks ranging from coding to content creation and imagery selection, thus enabling the rapid addition of new variants to experiments. As articulated by one of our partners, "Evolv AI enabled us to condense 6 years' worth of experimentation into just 3 months."
- Integrates: In today's digitally interconnected landscape, your data ecosystem holds immense importance. Integrating insights from additional data sources into a manual A/B testing program can escalate the complexity of analysis. However, this challenge is effortlessly managed with AI, which automates the process and handles the calculations on your behalf. By amalgamating data from multiple sources, AI paints a more comprehensive and nuanced picture, ultimately leading to actionable outcomes.
Developing and deploying A/B tests manually can be a time-consuming process, often taking developers months to build, particularly when upper management requests new features or functionalities at the last minute. However, leveraging AI enables you to utilize automation to generate experiments swiftly, allowing for more rapid testing with reduced risk. This approach aids in maturing an experimentation program at scale by streamlining the process and enabling quick adaptation to changing requirements.
Building Multivariate Tests with AI
Before initiating testing, it’s crucial to identify the metric you aim to optimize. Typical Key Performance Indicators (KPIs) for experimentation include enhancements in:
Average order value (AOV)
Units per order (UPO)
Sign-ups
Subscriptions
Checkouts
Bookings
Lifetime Value to Customer Acquisition Cost ratio
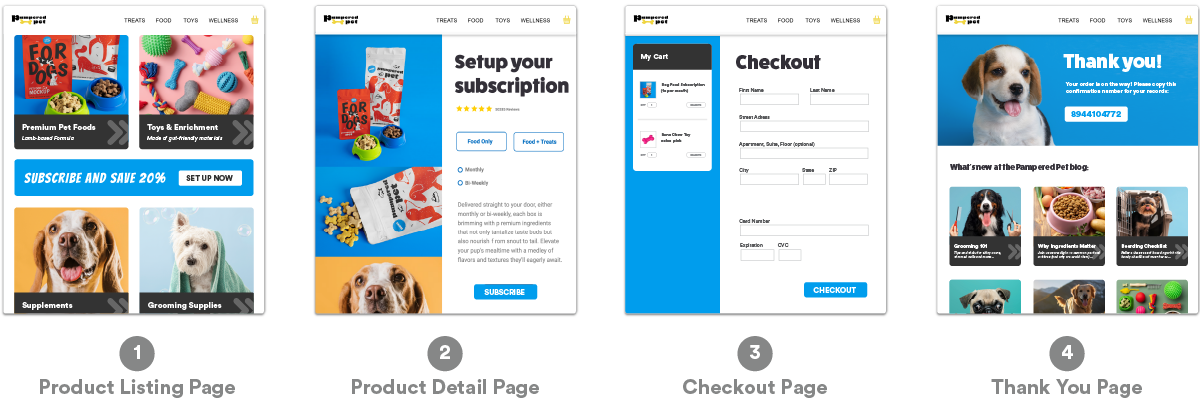
When aiming to optimize a KPI like “checkout completion,” it’s typically recommended to focus on the touchpoint within the flow that holds the highest significance or potential friction. For instance, the checkout page often assumes great importance because users have already committed to making a purchase – the challenge now lies in encouraging them to finalize the transaction.
Thus, let’s formulate a hypothesis to enhance the checkout page.
Your hypothesis can be informed by either data or intuition. For instance: “I suspect that our users may not be fully aware of the contents of their cart at the checkout page. To enhance performance, we propose adding detailed cart information throughout the entire checkout process, which we believe will lead to a higher rate of successful checkouts.”
You can leverage Evolv AI’s recommendation engine to receive suggestions for experiments likely to drive conversions.
Consider these additional questions to aid in forming your hypotheses:
What personal information is necessary and why? Can any questions be omitted for brevity? Should additional questions be included for security?
Are the fields requesting personal information logically ordered?
Do large purchases result in more drop-offs than small ones? How can consumer trust be established before soliciting personal information?
Are cart items prominently displayed?
Is the mobile viewport being fully utilized?
Are multiple calls-to-action present on the desktop version?
Are there actions beyond reviewing the order and proceeding necessary?
How much order information is visible? Should certain details be collapsed?
Have promotional options been applied on the product detail page (PDP), and are they visible in the cart?
Elements on the checkout page to test include:
Checkout button color and placement
Inclusion of trust symbols
Messaging adjustments for returning visitors
Addition of social media sign-up options
Offering a guest checkout option
Personalization of the cart
Messaging refinement for clarity
Reduction of multiple CTAs
Emphasis on free or expedited shipping and easy returns
Introduction of bundled products or "you may also like" carousels with one-click add-to-cart functionality
Clear display of promotions
You can utilize Evolv AI’s library of UX recommendations to kickstart your experimentation program. Continuous optimization will ensure the delivery of the best versions of experiences to the appropriate audience at the right time.
Moreover, you need not concern yourself with the tactical aspects of developing various asset versions for your experiments. With Generative AI, simply convey your prompt to Evolv AI’s assistant, Cali, to generate images, text, and code seamlessly within the experimentation platform.
Getting started with AI-led Experimentation
Machine learning algorithms may seem daunting for developers, but integrating them with Evolv AI is straightforward, offering a no-code to low-code experience.
All you need to begin with Evolv AI is a simple snippet added to the head of your site.
Key Performance Indicators (KPIs) such as Average Order Value (AOV), Units per Order (UPO), Sign-ups, Subscriptions, Checkouts, Bookings, and the Lifetime Value to Customer Acquisition Cost ratio are often directly linked to specific stages of the customer journey. For instance, the “checkouts” KPI is associated with a checkout flow, which encompasses elements like a product landing page, product detail page, checkout page, and a thank you page, marking a completed conversion.
Interested in learning more about Evolv AI? It’s the first AI-led experience optimization platform that recommends, builds, deploys, and optimizes customer experiences to impact behavior and drive business outcomes.